How to Prevent Blindness With AI?
How Google created a deep learning algorithm to detect diabetic retinopathy and applied it in rural India to combat blindness.
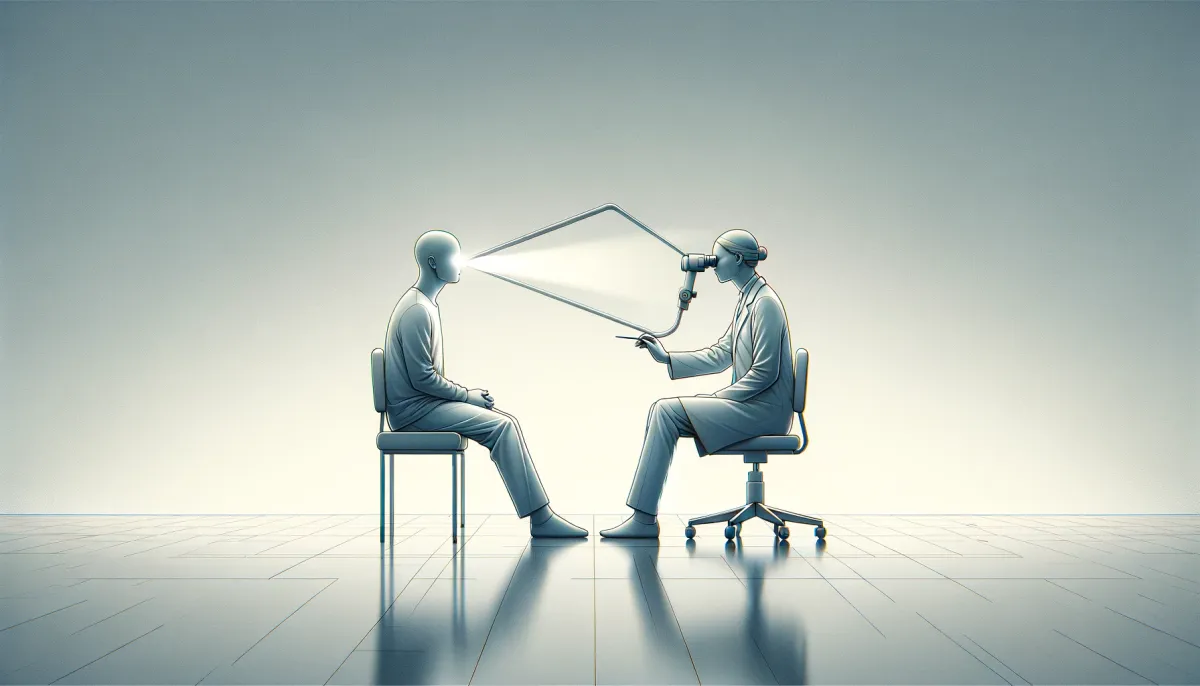
Almost 30 million Americans suffer from diabetes and its prevalence is steadily rising. One of its major complications, diabetic retinopathy (DR), is present in about 30% of diabetics. If left untreated, it leads to blindness.
To prevent it, diabetics are involved in yearly screening programs and checkups. However, we can only assume that the prevalence of diabetes and thus DR will continue to rise. And as stated many times before, the number of doctors simply cannot follow up to the demand. To illustrate the point, less than 10% of diabetics in India are screened for DR.
That's why AI and other digital technologies are on the rise and why countless studies are conducted on the topic. Google took on the challenge by funding one of the most impactful papers from 2016, boasting with almost 5000 citations and more than 153 highly influential ones since.
They wanted to show how DR screening could be automated with deep learning and (convolutional) neural networks. Achieving even some form of automation would be very positive for screening efficiency and reproducibility. Not to mention, it would significantly improve patient access.
Developing the algorithm
The study was similar to The Panda Challenge, except for the competition part. The researchers used 3 data sets - one for training the algorithm and two for clinical validation. Multiple ophthalmologists in turn graded all images for DR on a scale from 1 to 5.
A deep learning function separately graded the severity of DR based on intensities of the pixels on fundus images and used ophthalmologists' diagnoses to train itself.
Results
After training the algorithm on almost 130K fundus images and validating it on two separate image sets, the researchers determined its performance. They used different operating points, and evaluated its specificity and sensitivity for each.
In the case of screening for DL, it's crucial for the algorithm to have high sensitivity, which is also how the operating points were chosen. The end result was 97.5% sensitivity and 93.4% specificity.
The results indeed confirmed that deep neural networks can be trained to identify DR in retinal fundus images with high sensitivity and specificity.
Limitations and epilogue
The effectiveness of every algorithm is dependent on the data that we feed it. As a result, if the majority of ophthalmologists wouldn't be able to recognize the images, nor could the algorithm. Though unlikely, there's room for potential errors. Furthermore, deep networks learn by themselves, so it's possible the algorithm is using image features unknown to humans. Given that it's trained to only detected diabetic DR, it could also miss other (but similar) diseases, such as non-diabetic DR. It thus cannot fully replace a comprehensive eye examination.
The epilogue of this paper is especially interesting and exciting. After publishing it, Google partnered with its healthcare branch Verily to put theory into practice. In 2019, they launched a screening system in India, using the findings from this study and an application called ARDA (Automated Retinal Disease Assessment) to help prevent blindness caused by DR. They demonstrated that it is in fact possible to (partially) automate DR screening and apply it in a clinical setting. It certainly helps if you're Google.
